Research themes
Smart data, combined with traditional sources, can help researchers better understand a wide range of social and economic patterns and processes. Our research is divided into six categories.

Labour market
Our research examines how environments shape attainment and skills development, and how the job market and the nature of work are evolving.

Housing and neighbourhoods
Housing and Neighbourhoods examines where people choose to live, the housing they can access, and the qualities of their neighbourhoods.
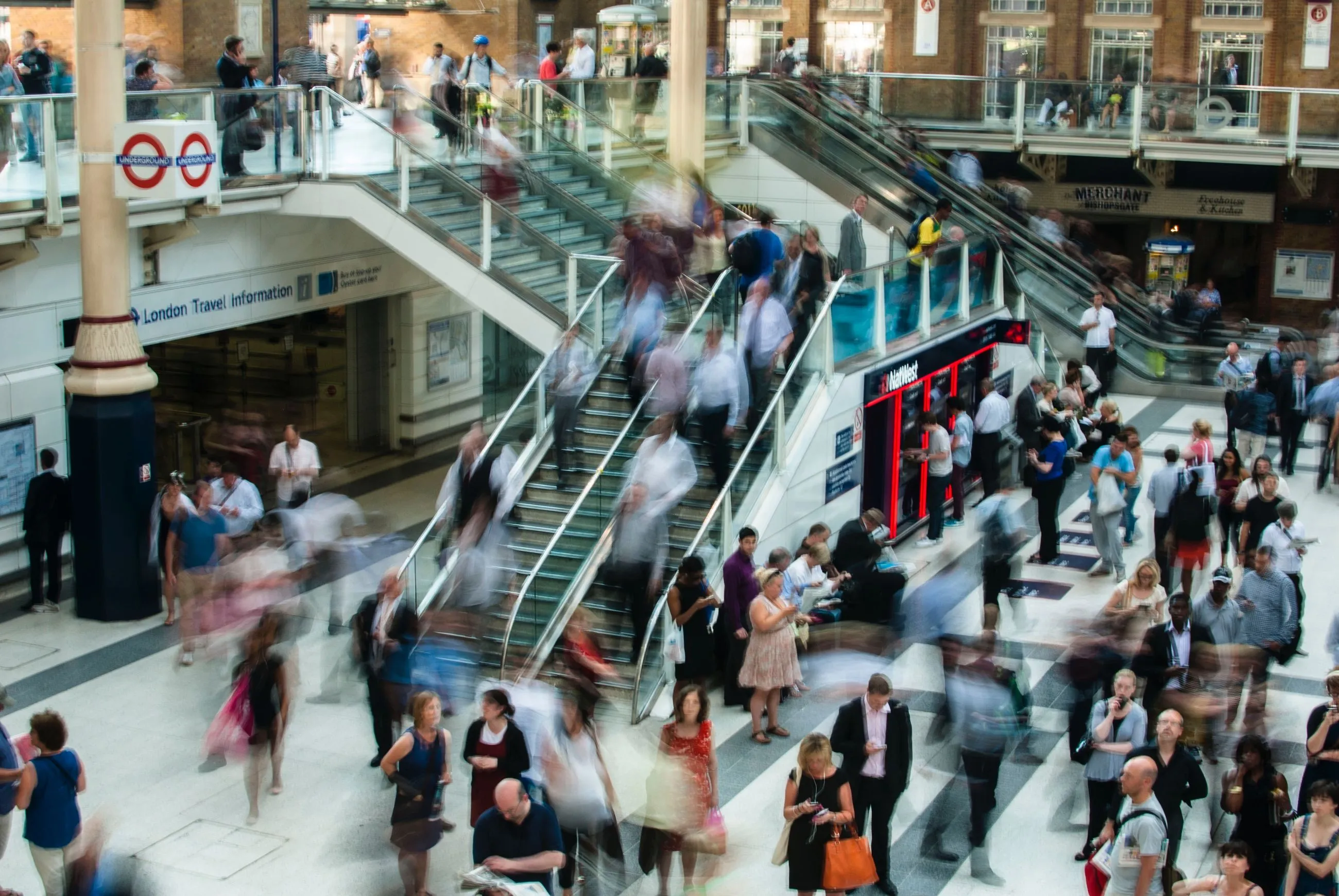
Transport and mobility
Transport is a key urban sector indicator and we use new forms of data to better understand travel behaviour in cities.
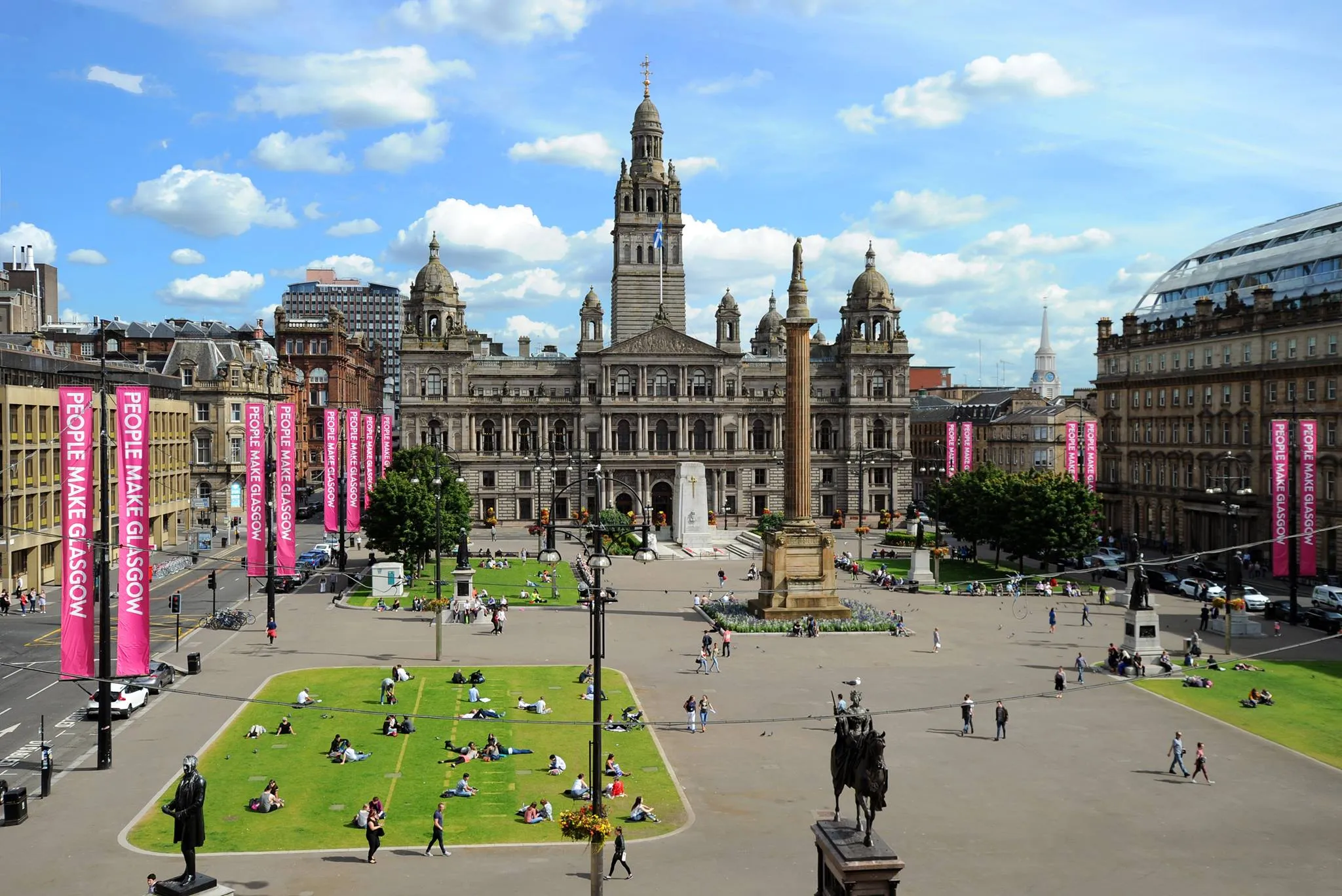
Urban governance
Our research investigates where and how smart data can shape city planning and policymaking on diverse urban issues, such as public transportation, waste management, and social service delivery.
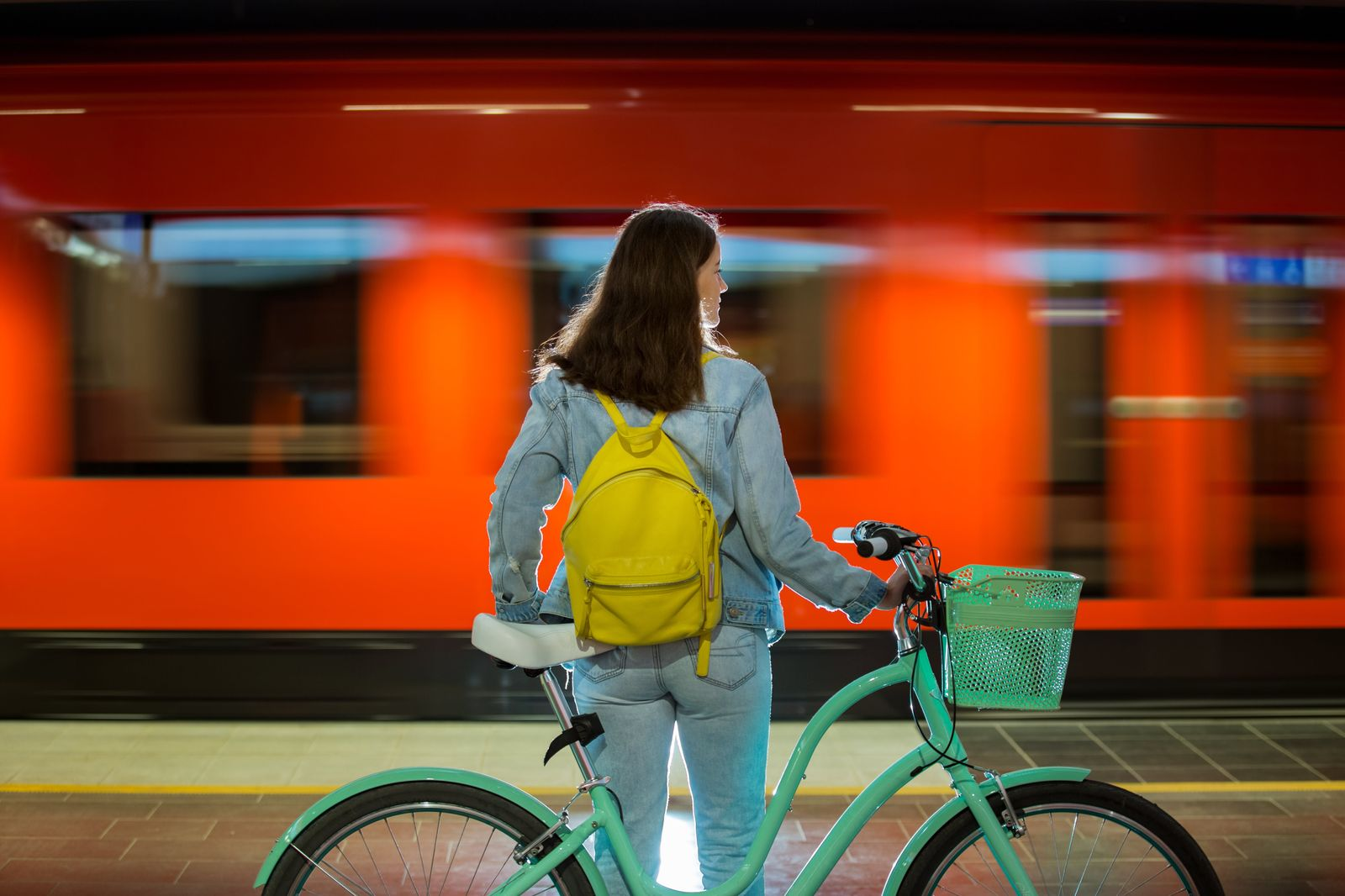
Urban sustainability
Our research concerns inclusive data innovations that support climate action and enable progress towards the United Nation’s Sustainable Development Goals.
.webp)
Education
We examine how urban educational systems shape attainment, skills development and productivity.
Jointly funded by
%20copy.png)
.png)